AI for Snowflake cost optimization
Based on years of cutting-edge research at top universities, Keebo’s patented technology is the only fully-automated optimizer
One-of-a-kind
Other Snowflake “optimization” products can only report, and cannot capture real-time savings opportunities or protect performance 24×7
Keebo: observe and act | Others: observe and report | |
---|---|---|
Real-time optimizations | Yes | Human required |
Real-time perf. protection | Yes | Human required |
User control | Set and forget | Human intervention required |
Pricing | Aligned on savings | Misaligned on spend |
Monitoring and visibility | Performance, usage, cost | Performance, usage, cost |
Approval workflows | No | Yes |
Verifiable, secured, controlled
We’re friendly robots, so we never leave you in the dark, and you create rules and conditions for confident set-it-and-forget-it operation
Pricing aligned with you
Keebo’s unique pricing ensures that our goals are aligned –
we don’t win unless you win
Option 1:
Keebo on commission
What you pay is based
on what you save
Option 2:
Keebo flat rate
All your optimizations
for a fixed price
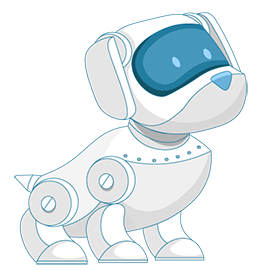

Try
Free trial, set up in only 30 minutes

Verify
Proposal with verified savings

Buy
Enjoy saving money and time!
FAQ
Setup takes about 30 minutes, and we will step you through it when you are ready to start a trial. You can create your own Keebo accounts or use Okta SSO. Keebo requires access to Snowflake’s usage metadata fields only, and you create the Snowflake account we use to access those fields.
Keebo’s Warehouse Optimization does not alter your queries. Instead, we constantly monitor and make dynamic optimizations to various aspects of your Snowflake settings, such as warehouse size, auto-spend time, and number of clusters. Importantly, Keebo protects your query performance by performing automated “backoffs” by reverting to your default settings, whenever there is a spike in your workload or query queues are increasing. Further, you can monitor and control all of this as much as you want, or set it and forget it.
Keebo’s patented technology is the only automated Snowflake optimizer. Other products can only observe your Snowflake systems and then give you reports and sometimes recommendations, leaving you to make the final decisions on which recommendations to implement. But workloads are dynamic, which means you can miss significant savings opportunities if you are not available around the clock. Further, if an optimization later causes query latency, you also need to be around to fix it. Unless you have the luxury of sitting in front of Snowflake 24×7, you should use Keebo instead of one of these “observe and report” products. You can learn more about the difference here.
Even though Keebo is capable of fully automating optimization, it is never a black box. You are always in charge and have fine-grained control over which optimizations will be applied to each warehouse. You also have full control over the timing of the optimizations. In addition, you can see and verify Keebo’s optimizations as well as audit the actions your team members have taken directly in our user portal.
By default, Keebo only finds optimizations that are very unlikely to negatively affect query latency. We monitor each warehouse’s performance, and if necessary, we will “backoff” our optimization to ensure performance. Additionally, you can change the settings to make our algorithms more aggressive to save more money, or you can choose more conservative settings to make performance slowdowns nearly impossible. You control this for each warehouse using our intuitive slider bar.
Let’s get started
“I went from ‘this sounds too good to be true’ to ‘this is a no brainer’ after one week of trial.” – Director of BI, Team Velocity
See it in action
View our collection of demo
videos.
Keebo for Snowflake
Get the definitive guide on using AI to reduce Snowflake costs.
Talk to our experts
Get a personalized demo or free trial.